How to Balance AI Detection Benefits With the Risk of False Positives
Artificial Intelligence (AI) detection tools are revolutionizing various industries, but their implementation comes with both benefits and challenges. This article delves into the crucial balance between leveraging AI detection capabilities and mitigating the risks of false positives. Drawing on insights from experts in the field, readers will discover practical strategies for effectively integrating AI tools while maintaining human oversight and judgment.
- AI Essay Grader: Guide, Not Gatekeeper
- Choose Reliable Tools, Communicate with Users
- Calibrate AI Detection with Human Oversight
- Combine AI Patterns with Human Judgment
- Refine AI Through Testing and Feedback
AI Essay Grader: Guide, Not Gatekeeper
As the creator of the AI Essay Grader tool, I understand the stress that can arise when AI begins to "detect" your writing—no one wants their solid work to be flagged by a machine. That's why we've designed our AI-detection feature to be more of a helpful guide than a rigid gatekeeper.
First, every essay receives a simple "AI-likelihood" score—think of it as a heads-up display rather than a final grade. When that score exceeds our conservative 30% threshold, it moves the essay into a review queue for a teacher. A human then examines the context, tone, and intent—no one ever loses points without another human taking a second look.
Teachers can also see which sentences raised concerns and ask the student if they feel it's a false alarm ("I actually quoted a class text here," or "I was trying out a new style"). Behind the scenes, we're constantly testing our model—mixing in purely human and AI-generated essays to adjust our settings and keep false positives below 1%.
In short, our mission is simple: detect actual abuse without ever penalizing legitimate work. By pairing smart algorithms with clear explanations and human oversight, we make AI detection a trustworthy sidekick, not a scary black box.
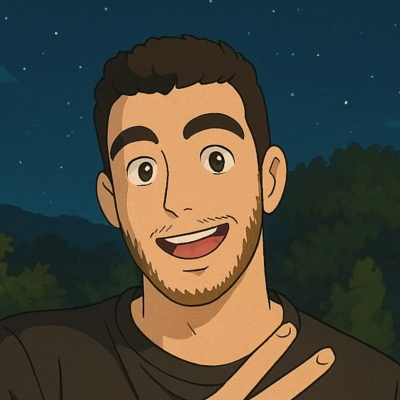
Choose Reliable Tools, Communicate with Users
It all starts with choosing a reliable detection tool. Some are simply better than others in terms of accuracy and their ability to detect various AI language models. Also, depending on how or what you are using the detection tool for, it can be wise to ask the person who provided you with the work that your tool detected as potentially AI-generated. If you are a teacher, for example, to give your student the benefit of the doubt, if your AI-detection tool highlights potential AI use, it's advisable to talk to your student about it first before simply giving them an F.
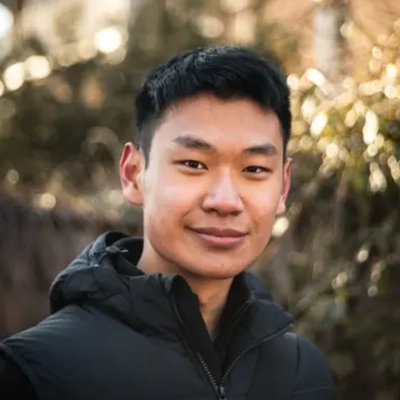
Calibrate AI Detection with Human Oversight
Balancing the potential benefits of AI detection with the need to avoid false positives and maintain fairness comes down to careful calibration and constant monitoring. AI can be a powerful tool for detecting fraud, misconduct, or inaccuracies, but if it's too sensitive, it can flag harmless actions, creating unnecessary complications. On the flip side, if it's not sensitive enough, it can miss real issues.
For example, in a previous project where we used AI to detect fraud in eCommerce transactions, we faced the issue of false positives, where legitimate customers were being flagged as fraudsters. To address this, we implemented a multi-tiered approach. First, we refined the AI model with better training data that accurately represented different types of customer behavior. Then, we built in a human review layer for flagged transactions, ensuring the AI's decisions were verified before taking action.
The key was finding the sweet spot: using AI to handle the heavy lifting while leaving room for human judgment to ensure fairness. Regularly reviewing the model's performance, analyzing false positives, and adjusting the algorithm as needed helped us strike that balance. This allowed us to catch fraud effectively without punishing legitimate customers.
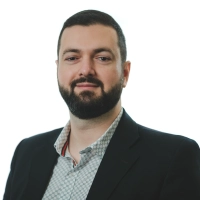
Combine AI Patterns with Human Judgment
Balancing the promise of AI detection with the risks of unfair labeling is a constant tightrope walk. In my work reviewing student essays for originality, I recall one incident where the system flagged a paper as likely AI-generated.
I knew the student struggled with English but was hardworking, so instead of jumping to conclusions, I read the essay closely. The style was a bit formulaic and lacked errors, which usually triggers suspicion. However, some awkward phrasing and genuine insight stood out, details that a basic language model tends to miss.
I reached out to the student and talked about her process. She explained how she used grammar checkers to revise but wrote every word herself.
At that moment, the value of adding a human layer to AI detection became clear to me. Technology can spot patterns, but it can't always read intent or context.
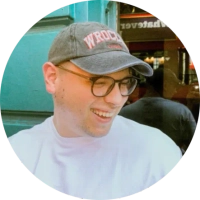
Refine AI Through Testing and Feedback
Balancing the benefits of AI detection with the need to minimize false positives and ensure fairness is a delicate endeavor. In practice, this often involves continuously tweaking the algorithms to improve accuracy while rigorously testing them across diverse scenarios. A real-life example of this is in the healthcare sector, where AI is used to detect diseases from medical images. Here, developers have to be extraordinarily careful because a false positive could mean an unnecessary invasive procedure for a patient, while a false negative could mean a missed diagnosis with potentially fatal consequences.
One approach to maintain this balance is the implementation of multi-stage testing phases where AI systems are first evaluated in controlled environments before being deployed in real-world settings. During these phases, feedback from end-users like doctors can be incorporated to refine the AI, ensuring it not only detects conditions accurately but also does so in a way that fits seamlessly into the existing workflow. Additionally, fairness is addressed by training these systems with diverse datasets that reflect different demographics, thus reducing the risk of bias against any particular group. Ensuring these measures might slow down the initial rollout of AI technologies, but they are crucial for maintaining trust and reliability in AI systems across various fields.
Conclusively, the path to achieving a harmonious balance between advantages and potential downsides in AI applications is ongoing and necessitates a thoughtful integration of human feedback, rigorous testing, and inclusive data practices. By doing so, we make significant strides in harnessing AI's potential responsibly.
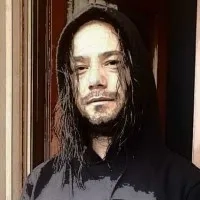