6 Strategies to Mitigate Bias in AI Detection Tools
Imagine a world where AI detection tools could be completely free from bias—sounds ideal, right? This pressing issue is addressed by industry leaders such as a Web3 SEO Agency Founder and a Social Media Expert, who share their expertise in our latest Q&A blog post. The discussion begins with the importance of prioritizing diverse training datasets and concludes with engaging multidisciplinary teams to tackle the challenge. With six expert insights in total, this article provides a comprehensive look at mitigating bias in AI detection tools.
- Prioritize Diverse Training Datasets
- Implement Bias-Detection Algorithms
- Ensure Data Quality and Diversity
- Audit and Update Data Sets
- Capture True Mix of Backgrounds
- Engage Multidisciplinary Teams
Prioritize Diverse Training Datasets
AI-detection tools, while powerful, often carry inherent biases due to the data they're trained on, which can skew results, especially in nuanced areas like tone, context, or cultural language variations. This can lead to misinterpretation, such as labeling perfectly valid content as AI-generated or misclassifying human-authored work.
To mitigate this risk, we need to prioritize diverse and representative training datasets that account for a range of writing styles, industries, and cultural nuances. Additionally, incorporating human-review layers in high-stakes contexts—where bias could impact reputation or credibility—is essential to catch potential misclassifications. Regular audits and transparency about training-data sources can also help to improve these tools, allowing businesses to trust AI-detection results while minimizing harmful biases.
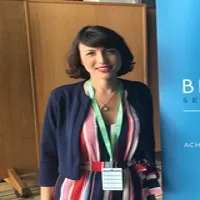
Implement Bias-Detection Algorithms
The potential for bias in AI detection tools is a significant concern, as these systems can inadvertently perpetuate existing biases present in their training data. For instance, if an AI model is trained on datasets that lack diversity or reflect societal prejudices, it may lead to skewed outcomes that disadvantage certain groups. This bias can manifest in various applications, such as facial recognition, hiring algorithms, or content-moderation tools, resulting in unfair treatment or misrepresentation of specific demographics.
To mitigate this risk, it is crucial to implement several strategies. First, using diverse and representative datasets during training can help reduce bias. This includes actively seeking data that encompasses a wide range of demographics, experiences, and perspectives. Additionally, incorporating bias-detection and correction algorithms can identify and address bias in real time. Regular audits and assessments of AI systems should also be conducted to evaluate their performance across different groups and contexts. Finally, involving a diverse team of stakeholders in the development and review process can provide valuable insights and help identify potential biases, ensuring that AI tools are more equitable and just in their applications.
Ensure Data Quality and Diversity
The potential for bias in AI detection tools is a significant concern, as these tools are increasingly used to make critical decisions in healthcare. Bias can arise from various sources, including biased training data, algorithmic design choices, and societal biases reflected in the data. This can lead to inaccurate diagnoses, discriminatory treatment, and limited access to care for certain populations.
To mitigate this risk, it is crucial to implement a comprehensive approach that addresses all stages of AI development and deployment. Here are some key strategies:
Data Quality and Diversity: Ensure that the training data used to develop AI detection tools is diverse, representative, and free from biases. This involves collecting data from diverse populations, including underrepresented groups, and carefully curating the data to remove any biases.
Algorithmic Fairness: Design algorithms that are fair and transparent. This includes using techniques like fairness constraints, counterfactual fairness, and algorithmic auditing to identify and address potential biases in the algorithms.
Continuous Monitoring and Evaluation: Regularly monitor the performance of AI detection tools to identify and address any emerging biases. This involves using techniques like demographic parity, equalized odds, and fairness metrics to assess the fairness of the tools.
Human Oversight: Incorporate human oversight into the decision-making process to ensure that AI decisions are fair and ethical. This involves training healthcare professionals to understand the limitations of AI and to critically evaluate the output of AI tools.
Transparency and Explainability: Make AI systems transparent and explainable to build trust and accountability. This involves providing clear explanations for AI decisions and making the underlying algorithms accessible for review and scrutiny.
Collaboration and Stakeholder Engagement: Collaborate with diverse stakeholders, including patients, clinicians, ethicists, and policymakers, to ensure that AI development and deployment align with ethical principles and societal values.
Audit and Update Data Sets
AI detection tools are powerful, but like any tool, they're only as good as the data they're trained on. Bias can sneak into these systems if the training data isn't diverse or balanced, which can lead to skewed results. As the founder of a chatbot, I see the importance of addressing this because biased AI can affect the user experience and even harm the brand's reputation. No one wants their technology to reflect unintentional bias—it's unfair and can create issues in how customers interact with our products.
To reduce this risk, I believe it's essential to frequently audit and update the data sets used to train AI tools. The broader and more diverse the data, the better the AI can perform fairly across different user groups. Another effective approach is incorporating human oversight—having diverse teams review AI decisions helps catch any bias the algorithm might miss, adding a layer of accountability.
Transparency is also key. By openly communicating how AI makes decisions and how data is used, we can build user trust and encourage feedback. It's a continuous process, but by taking these steps, we can create AI that's fairer, more reliable, and more aligned with the values of our users and our brand.
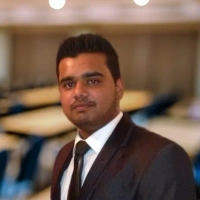
Capture True Mix of Backgrounds
Bias in AI detection tools is more than just a technical flaw—it affects people in real ways. When I worked with AI-driven analytics, I realized how even a slight imbalance in data could lead to unfair results. Imagine a dataset that's mostly from one demographic or focuses too much on certain patterns. Suddenly, the AI starts making assumptions that don't reflect everyone's reality. To make these tools fairer, datasets need to capture a true mix of backgrounds, languages, and experiences, bringing them closer to how we see the world.
It takes more than simply technological adjustments to create balanced AI; it also requires bringing together individuals with various viewpoints. Experts in psychology, sociology, and ethics can identify biases that a tech-only team would overlook. Frequent inspections also aid in identifying problems before they become serious ones.
Another important aspect of developing trust is being transparent with consumers about how these technologies operate. When people are aware that there is genuine effort being made to maintain fairness, their confidence increases. In the end, the goal is to develop AI in a way that values everyone's viewpoint.
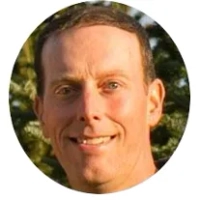
Engage Multidisciplinary Teams
The potential for bias in AI detection tools is a significant concern, as these tools can inadvertently perpetuate existing biases present in the training data. To mitigate this risk, it's crucial to ensure diverse and representative datasets during the training phase, incorporating varied demographics and contexts. Regular audits of AI systems can help identify and rectify biases over time. Additionally, implementing transparent algorithms that allow for human oversight and feedback can enhance accountability. Engaging multidisciplinary teams, including ethicists and sociologists, during the development phase can also provide diverse perspectives, ensuring that AI detection tools are fair and effective across different scenarios.
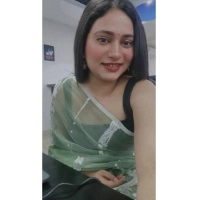